Building agentic AI in BFSI is so important for operations. Agentic AI offers financial institutions the ability to automate processes, personalize customer interactions, and optimize supply chains. This blog post outlines a practical roadmap for UK enterprises to successfully integrate agentic AI into their operations that 53% of the banks in the world have integrated AI chatbot to improve customer experience, now is the time to stay ahead of the competition.
Key takeaways:
✅ Lloyds Banking Group cut call center volume by 30%
✅ Aviva slashed claims processing from 5 days to 48 hours
✅ For every £1 invested, firms see £3.50 in ROI within 18 months (PwC)
Agentic AI in Financial Institutions for UK enterprise
The State of Agentic AI in BFSI
Agentic AI is rapidly redefining the landscape of the UK’s banking, financial services, and insurance (BFSI) sector. With 65% of UK banks now automating customer service through AI (Bank of England, 2024) and AI-powered fraud detection saving UK insurers an estimated £230 million annually (UK Finance), the benefits are tangible. Yurii notes the transformation is undeniable, citing success stories like Lloyds Banking Group, which cut call center volume by 30% using AI chatbots (Sweney and Jolly, 2025).
Even beyond the UK, innovations are emerging such as VeryPay, a fintech startup incubated at Verysell Group, where early Agentic AI in BFSI pilots in customer support have already demonstrated significant performance gains and operational cost reductions. However, amid the enthusiasm, a sobering reality persists: AI implementation remains challenging. Only 22% of firms have managed to scale agentic AI in BFSI solutions beyond pilot phases (McKinsey), and in 2025, the failure rate of agentic AI in BFSI projects surged to 42%, a 17% increase from the previous year (S&P Global Market Intelligence). These statistics underscore the need for a strategic and grounded approach to AI adoption—balancing the potential for ROI with a clear-eyed understanding of execution risks.
Why Agentic AI in BFSI?
Agentic AI in the BFSI sector (Banking, Financial Services, and Insurance) is emerging as a transformative force because it enables intelligent automation with greater autonomy, adaptability, and contextual reasoning than traditional AI systems. The rationale behind its growing adoption can be analyzed through the following compare-and-contrast table:
Aspect | Traditional AI in BFSI | Agentic AI in BFSI |
---|---|---|
Automation Scope | Executes predefined tasks (e.g., chatbot scripts, RPA) | Operates with goal-based reasoning, dynamically adapting to changing inputs |
Customer Experience | Limited personalization and reactive service | Delivers proactive, contextual, and hyper-personalized interactions |
Decision-Making | Follows rule-based logic or static ML models | Makes autonomous decisions based on multi-agent collaboration and real-time feedback |
Operational Efficiency | Reduces manual workloads in specific processes | Optimizes end-to-end workflows, enabling cross-functional process orchestration |
Scalability | Constrained by rigid logic and static data dependencies | Scales across departments with self-learning agents that improve over time |
Compliance & Risk Management | Supports compliance reporting through analytics | Proactively flags anomalies, mitigates risk, and explains decisions to regulators |
Innovation & Speed | Incremental improvements to legacy systems | Enables faster deployment of new financial products and services |
Examples | Static chatbots, fraud detection models | Autonomous credit underwriting agents, AI-powered compliance analysts |
Overall, agentic AI brings intelligence with agency-capable of not only processing tasks but also understanding goals, collaborating with other systems, and adjusting behavior to maximize value.
Top 5 ROI-Driven Use Cases
Fraud Detection
AI has significantly improved fraud detection accuracy by reducing false positives by 40%, which translates into an annual cost saving of £5 million for mid-tier banks. By minimizing the number of legitimate transactions mistakenly flagged as fraudulent, banks can enhance customer satisfaction while maintaining robust fraud protection, improving both operational efficiency and trust.
Hyper-Personalization
Through AI-driven analytics, financial institutions like HSBC have successfully deployed hyper-personalized product recommendations, leading to a 25% increase in cross-sell rates. By analyzing individual customer behaviors, preferences, and financial situations, AI tailors offers—such as bespoke mortgage packages at the right time, greatly enhancing customer engagement and conversion.
Claims Automation
Aviva has harnessed the power of agentic AI in BFSI to drastically reduce insurance claims processing time from five days to just 48 hours. This automation not only accelerates service delivery but also decreases administrative overhead, resulting in faster settlements and improved customer satisfaction in an industry where time-sensitive support is critical.
Customer Support
By embedding an AI chatbot directly within their app, one financial services provider managed to automate 75% of customer service interactions within just two weeks of deployment. This rapid implementation showcases the scalability and responsiveness of agentic AI, freeing human agents to handle more complex queries and ensuring 24/7 support for routine inquiries.
Credit Risk Assessment
One client struggling with the limitations of traditional credit evaluation methods turned to agentic AI in BFSI and achieved a transformative improvement. By using advanced algorithms, they could assess credit risk in mere seconds and enhanced predictive accuracy by 20%. This not only expedited decision-making but also expanded access to credit for previously underserved segments.
Challenges and Solutions
Data Silos
A major barrier to AI implementation in BFSI is the issue of data silos, with 58% of firms reporting difficulty accessing and integrating fragmented data across departments (Deloitte). These silos hinder the development of accurate, holistic models and slow down decision-making. To overcome this, our bespoke data unification frameworks consolidate disparate data sources into a centralized, structured environment, enabling AI systems to function with greater precision and consistency.
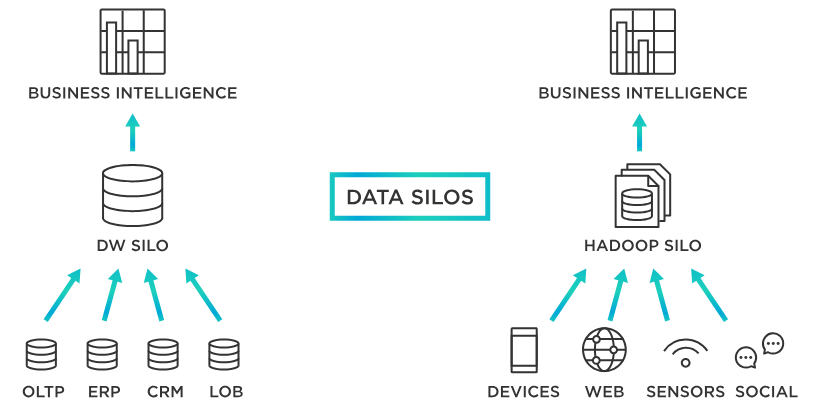
Regulatory Compliance
Another critical concern is regulatory compliance. Financial institutions must adhere to stringent frameworks such as GDPR and FCA guidelines, which demand transparency, fairness, and accountability in automated decisions. To meet these requirements, we implement explainable AI models that are inherently interpretable and fully auditable. This not only ensures legal compliance but also fosters trust among stakeholders by making AI-driven decisions understandable to both regulators and end users.
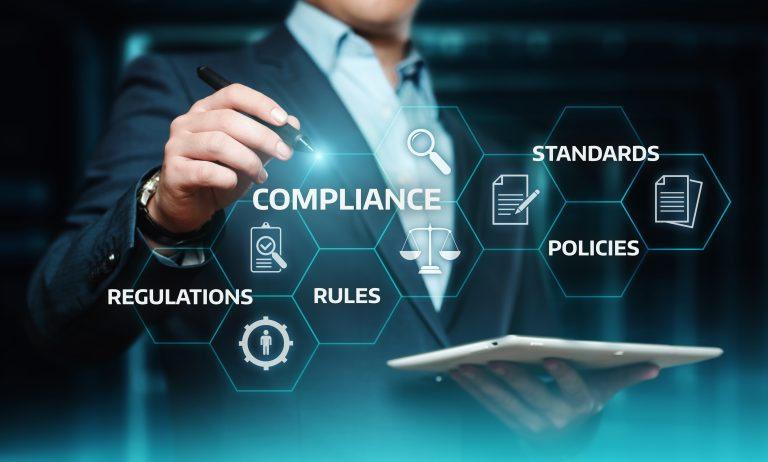
Legacy IT Infrastructure
Legacy IT infrastructure also presents a challenge, as many institutions operate on outdated systems not designed for advanced analytics or AI integration. Rather than requiring a full system overhaul, our API-first, modular AI deployment strategy allows organizations to embed intelligent capabilities within existing workflows. This flexible approach reduces implementation time, lowers costs, and ensures compatibility with core banking and insurance systems, making AI adoption both scalable and sustainable.
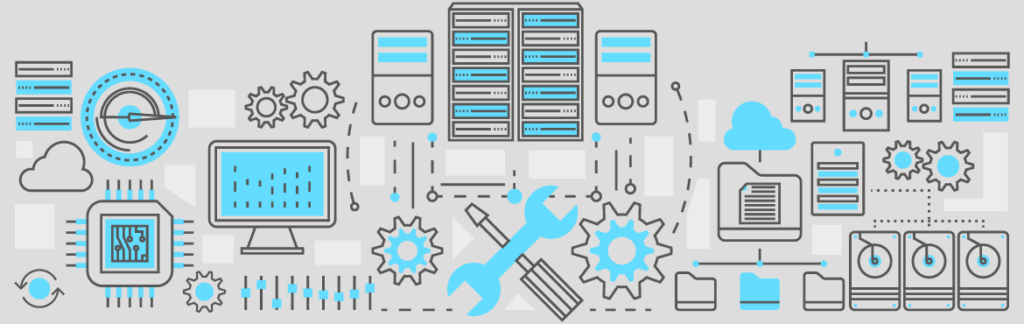
Roadmap to Adopting Agentic AI in BFSI
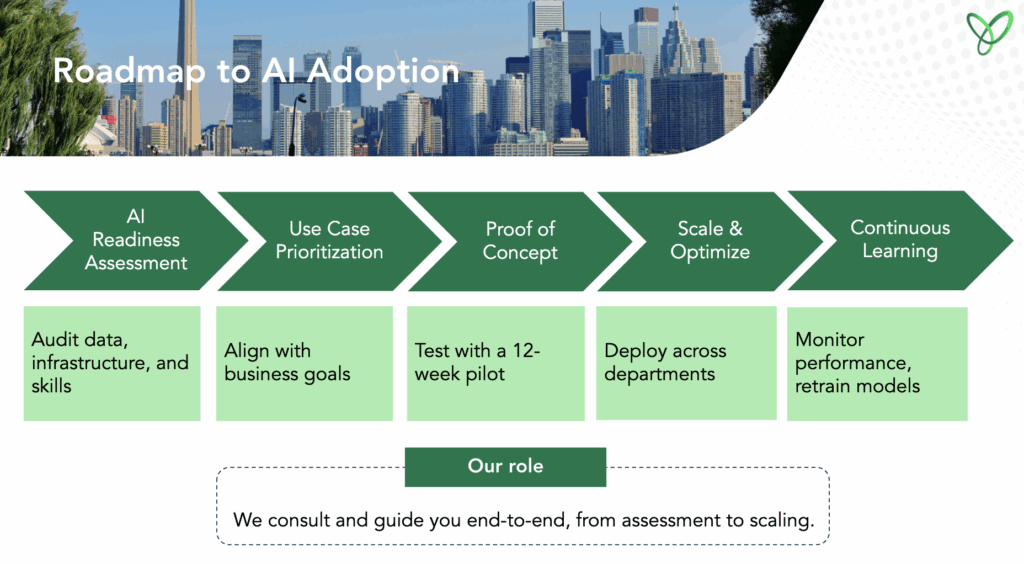
Conclusion
In this article, we explored how technology can enhance efficiency in the BFSI industry. Don’t forget to follow and contact us for the latest updates! We would love to hear about the webinar topics you are interested in for the upcoming sessions. Your feedback will help us create valuable and relevant content that meets your needs. Thank you for reading, and we hope to see you at the upcoming events!
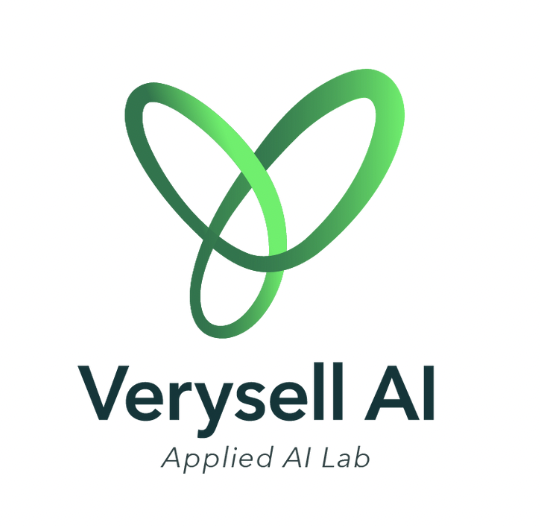