AI fraud detection and prevention in BFSI is the most famous use cases, which significantly enhances security measures in today’s world. Due to the growth of digital banking, implementing AI-driven solutions has become crucial for maintaining trust and safety in financial transactions. In this blog post, we will show more about AI fraud detection and prevention, as well as some of its use cases through malicious activity and the loss of finance in the banking industry.
>> Read more: https://verysell.ai/strengthening-financial-security-ai-in-fraud-prevention/
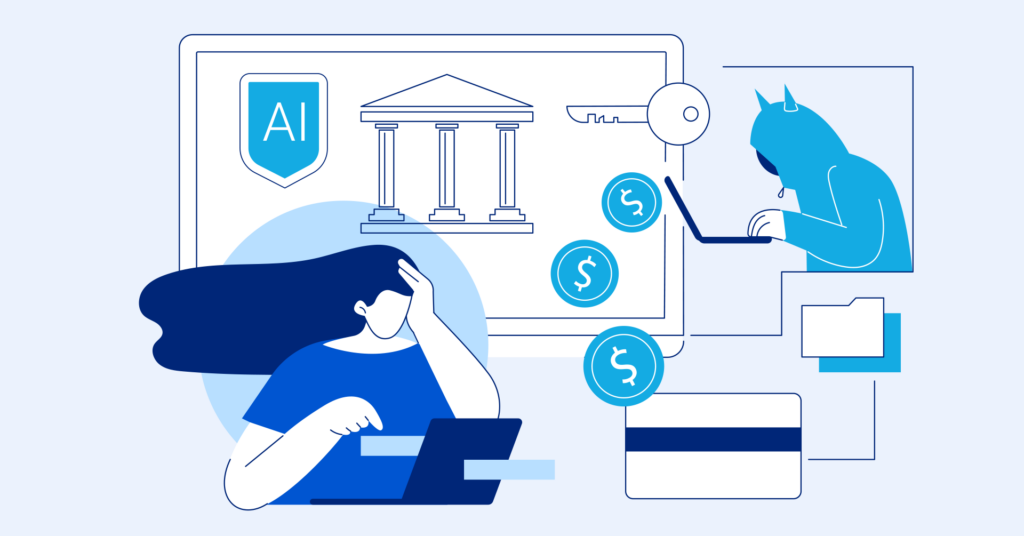
AI fraud detection and prevention in BFSI.
1. What is AI fraud detection and prevention?
AI fraud detection and preventation is a technology-based approach, employing machine learning to identify fraudulent activities within large datasets. It involves training algorithms to recognize patterns and anomalies that signal possible fraud. By continuously learning from new data, these machine learning models become increasingly adept over time, improving their predictive accuracy and enabling them to adapt to evolving fraudulent tactics to maintain transaction integrity and security.
For further insights, you can explore our LinkedIn post to discover the future of AI fraud detection and prevention in BFSI, discussing the role of AI in BFSI, shedding light on how similar technologies are applied in diverse financial sectors.
2. How does AI fraud detection work?
According to Signicat’s report The Battle Against AI-driven Identity Fraud, while over 76% of fraud decision-makers recognise the growing threat of AI in fraud, only 22% of organisations have started implementing AI-driven fraud prevention measures. AI fraud detection operates by implementing machine learning algorithms that are designed to analyze behaviors and detect anomalies indicative of fraud. Because the system encounters new and varied data, the AI model fine-tunes its parameters, differentiating between legitimate and suspicious activities more effectively.
The successful fraud detection systems leverage four key functionalities step-by-step: identity theft identification, which monitors unusual behaviors to protect consumer accounts; real-time transaction enabling immediate analysis of transactions for quick response; money laundering detection to identify suspicious patterns that indicate illicit financial activities and automated claims fraud detection be successful to protect the business. Together, these functionalities create a robust framework that enhances security and minimizes financial losses for organizations and consumers alike.
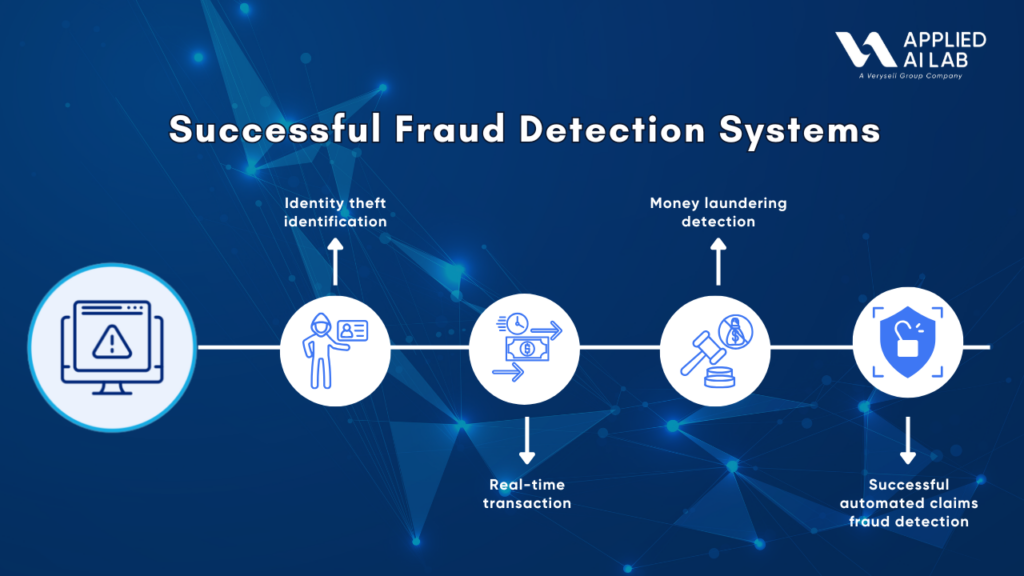
Succesful AI fraud detection systems
3. Use cases of AI fraud detection and prevention
3.1 Identity theft identification
Identity theft identification is used in biometric authentication for verifying processes that help significantly reduce the risk of identity theft. TransUnion 2024 shows that the United States experienced a 15% rise in data breaches between 2022 and 2023, highlighting the growing threat presented by hackers.
There are some signs of identity theft like: you see withdrawals from your bank account that you cannot explain, you find unfamiliar accounts or charges on your credit cards, and so on. By utilizing advanced AI in biometrics such as fingerprint recognition, face recognition, behavior recognition, and so on banks can ensure the authorization of the account to access private user information and transactions. The identification of theft not only enhances security but also optimizes user experiences, allowing customers to access their accounts fast and correctly while reducing the potential of unauthorized access.
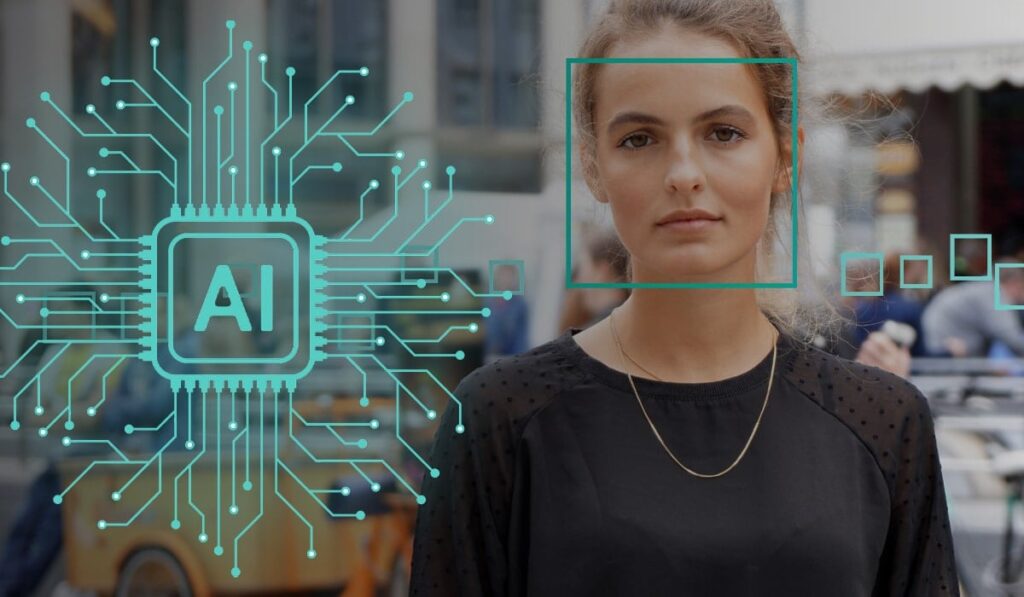
Facial recognition
3.2 Real-time transaction
AI systems help banks identify the transaction data in real-time, detecting unusual transactions that can affect the frequent activities of users. For real-time fraud detection, it exploits the mistakes in any fast transfers or various transactions in the short period. To do that, AI can research the amounts, frequency and mostly locations to show the basic behavior of customers.
When transactions is created in an unfamiliar location, the systems can create alerts for further investigation to protect users. This real-time capacity not only enhances methods to identify and prevent fraud but also helps the security of banking operations to gain the customer’s trust. You can monitor transactions by using tools like IBM Safer Payments to identify high-risk patterns faster and detect fraud with greater speed in many accounts or countries. AI models can analyze transaction data to detect behaviors like frequent small deposits by large withdrawals.
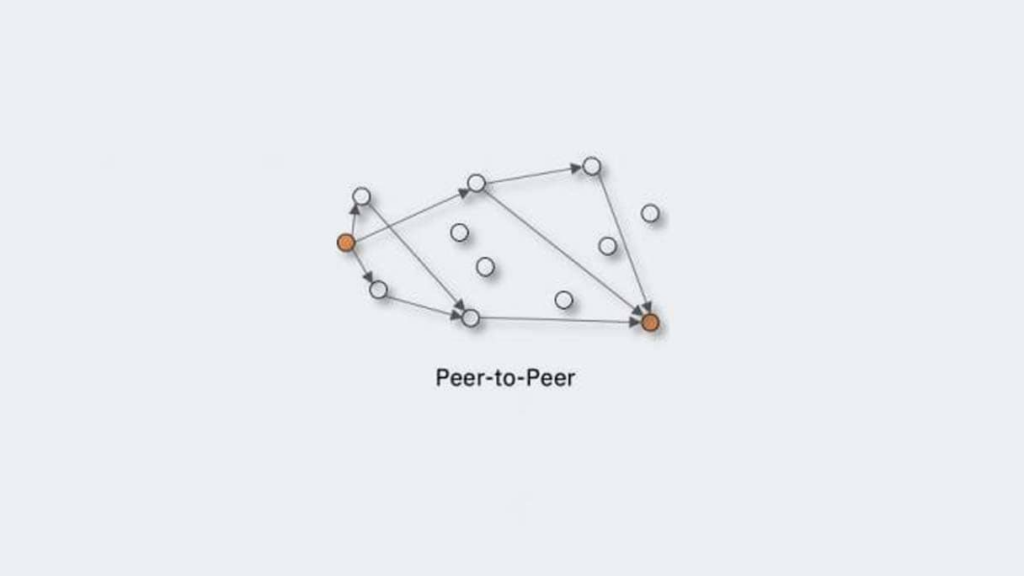
Real-time payments fraud prevention.
3.3 Money laundering detection
Without money laundering, drug cartels would have struggled to change their illicit proceeds into legitimate assets and expand their operations. According to McKinsey & Company, money laundering is processing through three steps: placement (bring money into the financial system); layering (conceal the origin of funds); and integration (move funds bank into the system using legitimate means).
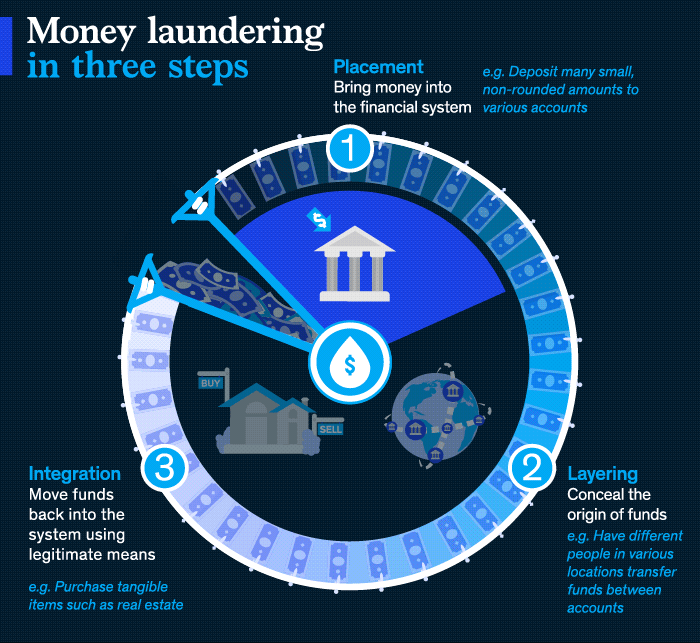
Money laundering in three steps.
Without money laundering, drug cartels would have struggled to change their illicit proceeds into legitimate assets and expand their operations. From that, deep learning models can identify hidden relationships between account behaviors and criminal actives, indicating money laundering those conventional methods. Therefore, companies can apply advanced systems to uncover suspicious activities that improve the overall detection rates and are more effective to avoid anti-money laundering efforts.
3.4 Automated claims fraud detection
AI automates claims assessment, catching fraudulent claims that manual processes might miss. By leveraging advanced algorithms and machine learning, banks can identify suspicious activities in real time, reducing fraud risks of financial losses that saves resources, reduces false claims, and protects businesses from financial losses.
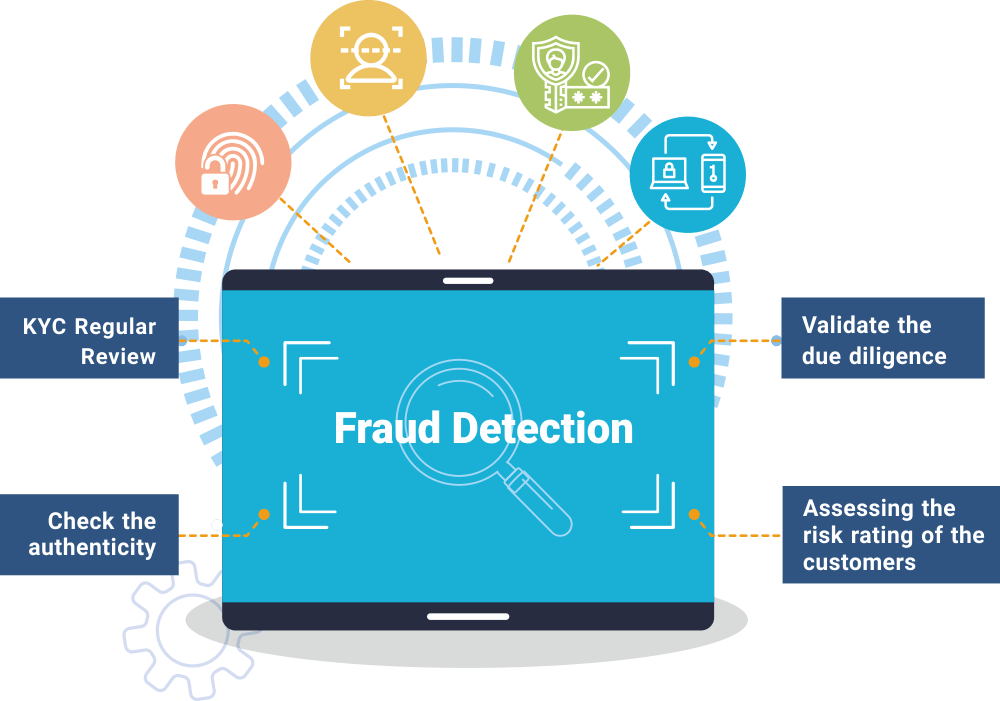
Automated claims fraud detection by leveraging AI.
For instance, AI-driven fraud detection solutions have been shown to reduce fraud-related costs by 30% to 50%, leading to substantial cost savings for businesses based on a study by McKinsey and Company. As technology continues to evolve, the potential for AI to refine fraud detection methods will only increase, further protecting financial institutions and their customers.
4. Conclusion
Our company, Applied AI Lab has a process to investigate financial fraud by using generative AI with 6 stages: data collection, feature engineering, model training, anomaly detection, deployment and monitoring and adaptation and improvement given by consulting experts. Therefore, when it comes to fraud detection in banking, we can easily see that AI services have supported minimizing banking thefts to reduce the loss of operations and enhance customer experience. From that, applying AI driven fraud detection solutions, banking institutions can enhance their fraud detection capabilities effectively.
Embrace the power of AI in claims fraud detection and join us in shaping the future of banking industry. Got a question about AI development? Talk to our expert!