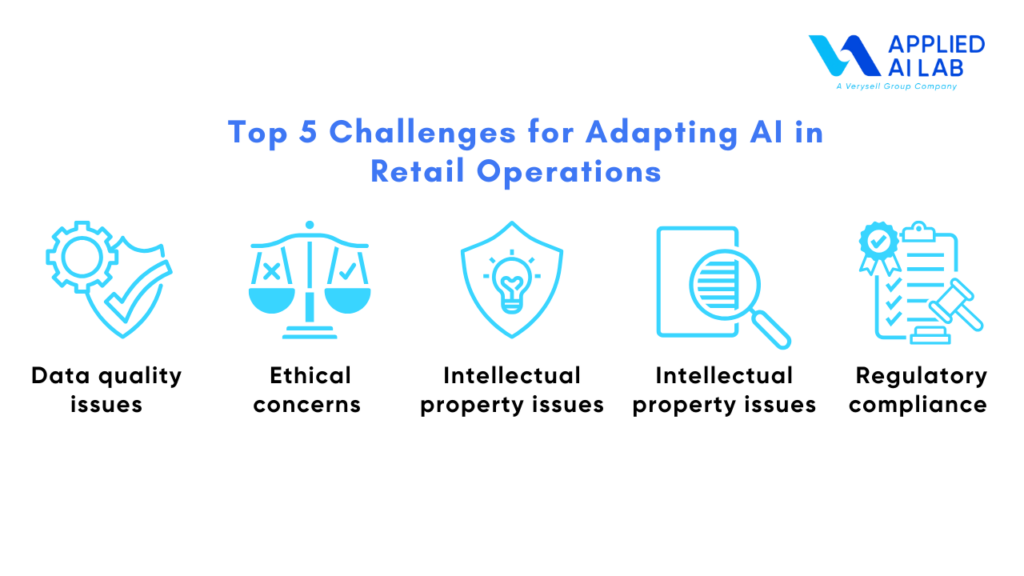
AI in retail operations has and will continue to revolutionize as other industries in the market like banking, insurance, financial services, and so on. According to PwC’s report, it is forecast that AI can potentially gain 15.7 trillion dollars to the global economy by 2030. In this blog post, we will discuss the top five barriers of adapting AI in retail operations such as data quality issues, ethical concerns, intellectual property issues, regulatory compliance and transparency in AI. Therefore, business leaders should know what they are and how to harness AI’s potential efficiently.
>> Read more: Top 4 Best Agentic AI Benefits in Retail Operations
Top 5 AI in Retail Operations Damaging Challenges
1. Data quality issues of AI in retail operations
One of the most important values when integrating AI is high-quality and available data, which is the foundation of any AI system. Thus, when data quality has any issue, it can affect the business’s growth.
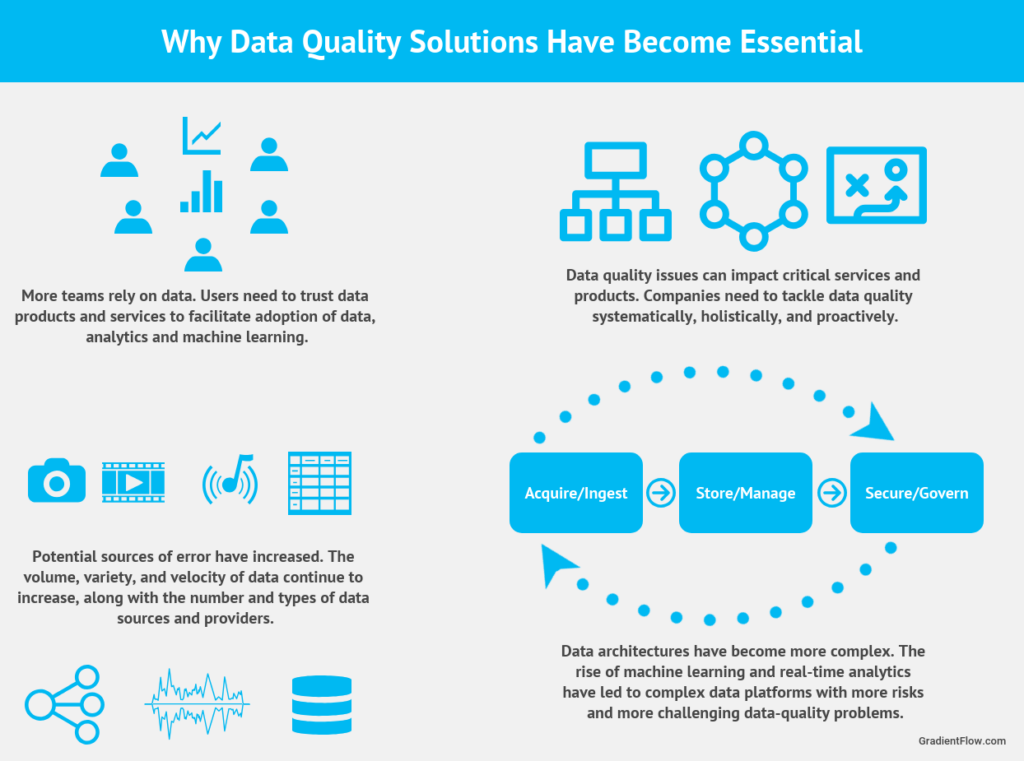
Data quality solutions have become essential in the AI market.
1.1 Data quality over quantity
The quantity and quality of data impact the accuracy of outputs, so poor quality data can create unreliable outsources to the organization. These unreliable data can affect business operations, take Unity Technologies as an example, its losses of $110 million. While time-consuming and intensive resources, rigorous data cleaning and standardized methods are essential for protecting AI quality. Moreover, according to Gartner’s, poor data quality costs companies a average $15 million lost annually.
How to avoid: The comapny can establish a robust data governance framework to oversee data management practices, ensuring accountability and compliance. As well as, they can utilize monitoring tools to track data quality over time and establish feedback loops for continuous improvement.
1.2 Data privacy and security
AI data integration also has a potential challenge about privacy and security. AI systems integrate some concerns about privacy such as: data confidentially, regulatory compliance, and so on. Therefore, implementing AI data integration needs robust security frameworks, encryption protocols, and careful governance policies to mitigate these risks.
How to avoid: The company can start implementing strict access controls to limit data access only to authorized personnel, minimizing the risk of data breaches. Due to that, they can reduce the lack of privacy and security for data when applying AI.
1.3 Data accuracy
Errors and inaccuracies in data can lead to unreliable outcomes in AI models. When flawed data is used for training, the model learns these inaccuracies, which can result in incorrect predictions. This is particularly critical in fields like healthcare and finance, where mistakes can have severe consequences. Additionally, non-representative training data can cause biases, limiting the model’s effectiveness. To address these issues, organizations should prioritize data quality through rigorous validation and regular audits.
How to avoid: Business leaders can regularly evaluate the quality of data through validation checks to identify and rectify errors early, and utilize automated tools for data cleaning to efficiently remove duplicates.
1.4 Data timeliness
Outdated data can make AI models obsolete, as they depend on current information for accurate insights. When models are trained on stale data, their predictions and recommendations may no longer reflect real-world conditions. To maintain relevance, organizations must regularly update their datasets and retrain their models.
How to avoid: Businesses can start using automated data pipelines to streamline the process of data collection and integration, making it easier to access fresh data. They also can continuously monitor the performance of AI models to identify when they start to drift from expected outcomes in the company.
2. Ethical concerns in AI
Ethical standards are at the core of effective AI when people understand how AI systems help make decisions and deliver unbiased results. AI ethics refers to a set of values and techniques that widely accepted standards right or wrong of operations. If the company lacking ethical AI is a big misstep that creates biased algorithms, personal freedoms and mistrust. Addressing the ethical considerations in AI is morally imperative to build trust for using systems, so having guidelines is essential for developing AI at the same time to the safety of society.
How to avoid: Having guidelines from leaders outline acceptable behaviors and standards for decision-making through 6-stage-process. Beside, ensuring transparency in AI systems by clearly communicating how decisions are made and what data is used, fostering trust among users.
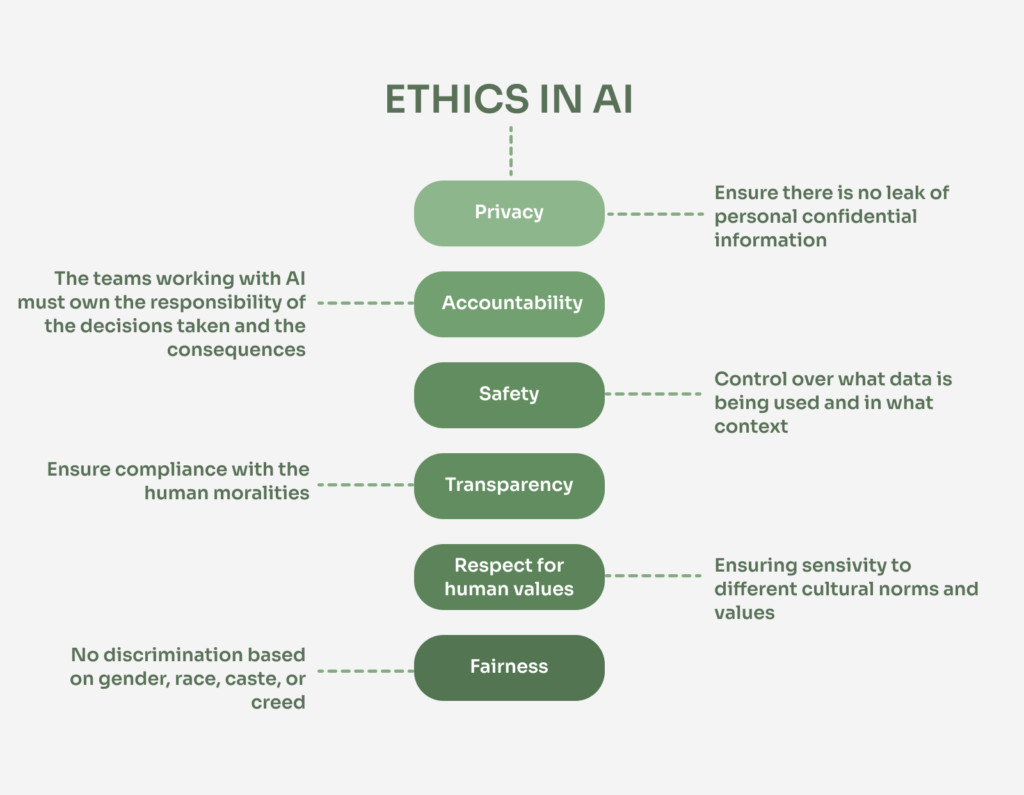
3. Intellectual property issues in AI
Intellectual property issues in AI can be complex, multifaced and affect retail operations in many sectors, with three ones: copyright, trademarks and patents. The first is copyright when utilizing AI-generated content for the business. In any process of retail operations, it is important to identify the licensing of AI to avoid infringement. Secondly, it is trademarks when retailers using AI for branding, such as generating logos or marketing slogans, must ensure these elements do not infringe on existing trademarks. Lastly, retailers developing proprietary AI technologies or systems may seek patent protection for their innovations. In this case, it can consist of unique algorithms or processes that enhance retail operations.
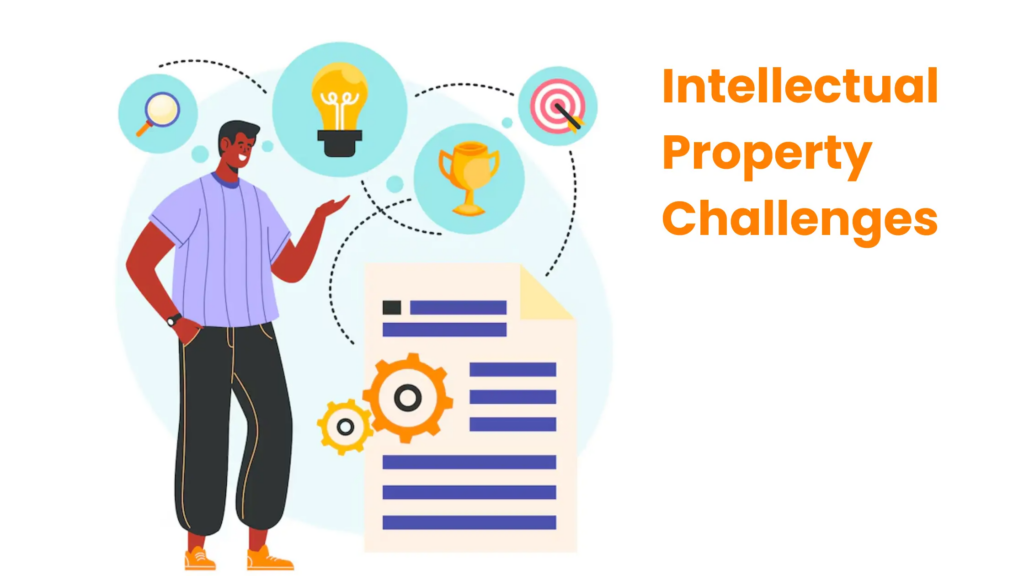
How to avoid: Organizations should ensure clear licensing agreements for AI-generated content to prevent copyright infringement. Conduct thorough trademark searches before using AI-generated branding elements to avoid conflicts with existing trademarks. Consulting legal experts can help navigate the complexities of intellectual property law when developing proprietary AI technologies.
4. Transparency in AI
Transparency in AI ensures fairness, accountability and trust to know how the systems work, which data they should use for their retailers, ensuring the standards of operations. Prioritizing trust and transparency with AI are essential for the business that involves documenting the decision-making process and making it more relevant to stakeholders. Besides, having clear communication is essential for managing the conversation between retailers and customers. From that, the bridge between intricate algorithms and human comprehension is crucial for maximizing AI’s potential in retail operations.
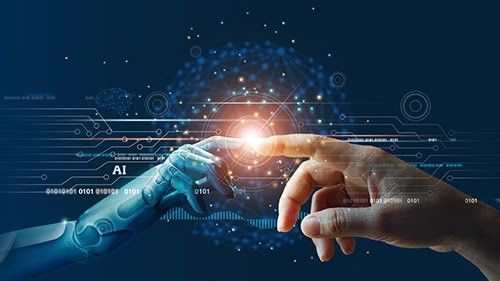
How to avoid: To avoid transparency in AI, organizations should document and clearly communicate the decision-making processes behind AI systems, ensuring stakeholders understand how data is used. Additionally, fostering open dialogue with customers about AI operations can help build trust and enhance understanding of the technology’s impact on retail.
5. Regulatory compliance
According to KPMG, 78% of consumers are stated that the organizations using AI have responsibilities to ensure its building ethically. AI compliance refers to the decisions and practices enabling businesses to stay in line with the laws and regulations of retailers that govern AI systems. AI compliance processes help businesses avoid the financial, legal and reputational risks associated with the use of AI tools.. Therefore, when AI technology develops, doing regulations governing helps retailers inform about changes in the legal landscape to ensure compliance.
How to avoid: Business leaders should establish a robust framework for monitoring and adapting to relevant laws and regulations governing AI use in retail. Regular training for employees on compliance requirements can help ensure everyone understands their responsibilities and the implications of non-compliance. Additionally, engaging with legal experts can provide guidance on navigating the evolving legal landscape and implementing necessary changes effectively.
Conclusion
As a part of Verysell Group, we specialize in creating tailored AI solutions for industries including banking, finance, insurance, e-commerce, and retail. Therefore, we believe that with our companion implementing AI carefully in the retail operations, retail operations can harness AI’s potential effectively. As AI continues to evolve, proactive management of these challenges will be crucial for success in the retail sector.