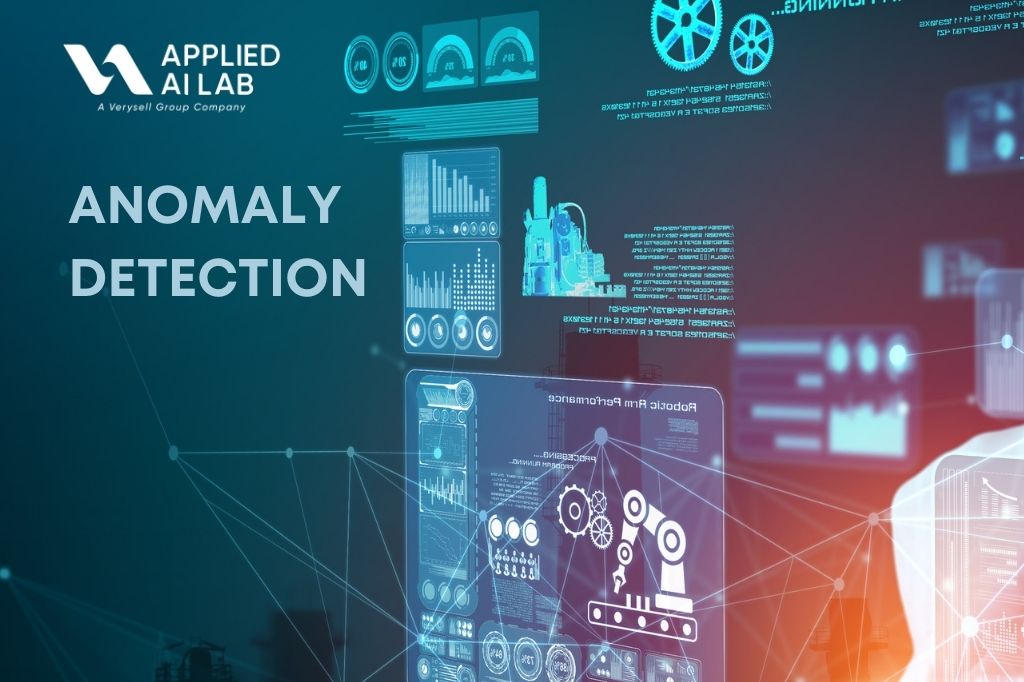
Business Challenges
For manufacturing businesses, particularly in industries reliant on heavy-duty machinery like power plants, steel plants, power transmission lines, and aviation, the continuous and uninterrupted operation of these assets is crucial. Any abrupt stoppage can result in significant financial losses and, more alarmingly, pose grave risks to human lives. Detecting early signs of anomalies in real time has, thus, emerged as a paramount need to avert such disruptions.
Traditionally, addressing this challenge has relied on periodic maintenance schedules and manual inspections. However, this approach has its downsides. It is often reactive, responding to problems after they have already occurred, leading to costly downtime and potential safety hazards. Additionally, adhering strictly to maintenance schedules can be wasteful when equipment may not require it.
This is where the application of AI, specifically Anomaly Detection in Data Analytics, has come to the fore as a groundbreaking solution. Leveraging advanced algorithms and data analytics techniques, manufacturing companies can now proactively identify deviations from normal equipment behavior. This proactive approach enables them to detect issues long before they escalate into full-blown failures.
The integration of Anomaly Detection into data analytics offers multiple benefits. It not only minimizes downtime but also facilitates predictive maintenance, optimizing asset performance and extending their operational lifespan. By analyzing vast amounts of data in real time, AI can pinpoint anomalies and irregularities that human operators might miss. Moreover, AI systems can continuously learn and adapt, improving their accuracy over time. This transformative force is safeguarding both economic interests and human well-being in these critical industries, ensuring that machinery operates smoothly, efficiently, and safely.
AI Solution
The implementation of the Attention-CNN-LSTM model yielded highly promising outcomes:
- Exceptional Accuracy: The model achieved a remarkable accuracy rate, nearly 99%, in detecting the three states of power transformers. This precision is vital for field engineers to make timely decisions regarding maintenance schedules.
- Proactive Maintenance: By understanding the timing of these states, field engineers can proactively schedule maintenance, reducing the risk of sudden power transformer failures.
- Operational Continuity: This proactive approach ensures uninterrupted operations, minimizes risks, and safeguards against potential financial losses and safety hazards.
In conclusion, this case study exemplifies the transformative potential of advanced AI solutions in anomaly detection for heavy-duty machinery. By leveraging AI technologies, industries can enhance operational efficiency, reduce risks, and prioritize safety, ultimately leading to more robust and resilient operations.
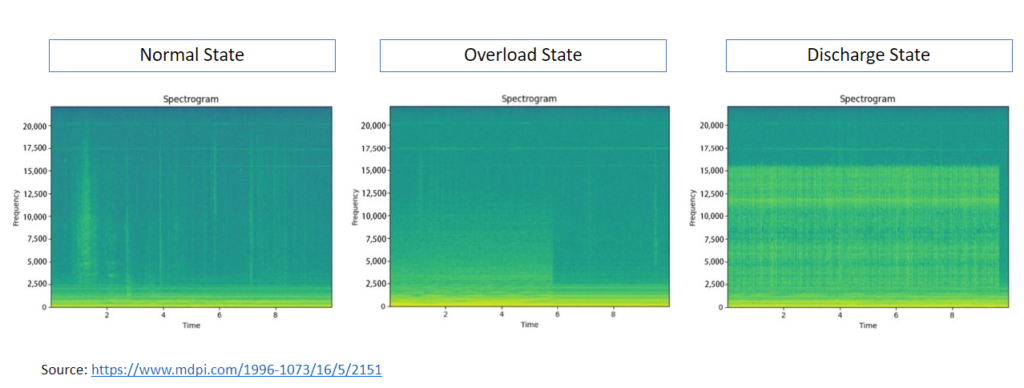
Expected Outcome
The implementation of the Attention-CNN-LSTM model yielded highly promising outcomes:
- Exceptional Accuracy: The model achieved a remarkable accuracy rate, nearly 99%, in detecting the three states of power transformers. This precision is vital for field engineers to make timely decisions regarding maintenance schedules.
- Proactive Maintenance: By understanding the timing of these states, field engineers can proactively schedule maintenance, reducing the risk of sudden power transformer failures.
- Operational Continuity: This proactive approach ensures uninterrupted operations, minimizes risks, and safeguards against potential financial losses and safety hazards.
In conclusion, this case study exemplifies the transformative potential of advanced AI solutions in anomaly detection for heavy-duty machinery. By leveraging AI technologies, industries can enhance operational efficiency, reduce risks, and prioritize safety, ultimately leading to more robust and resilient operations.