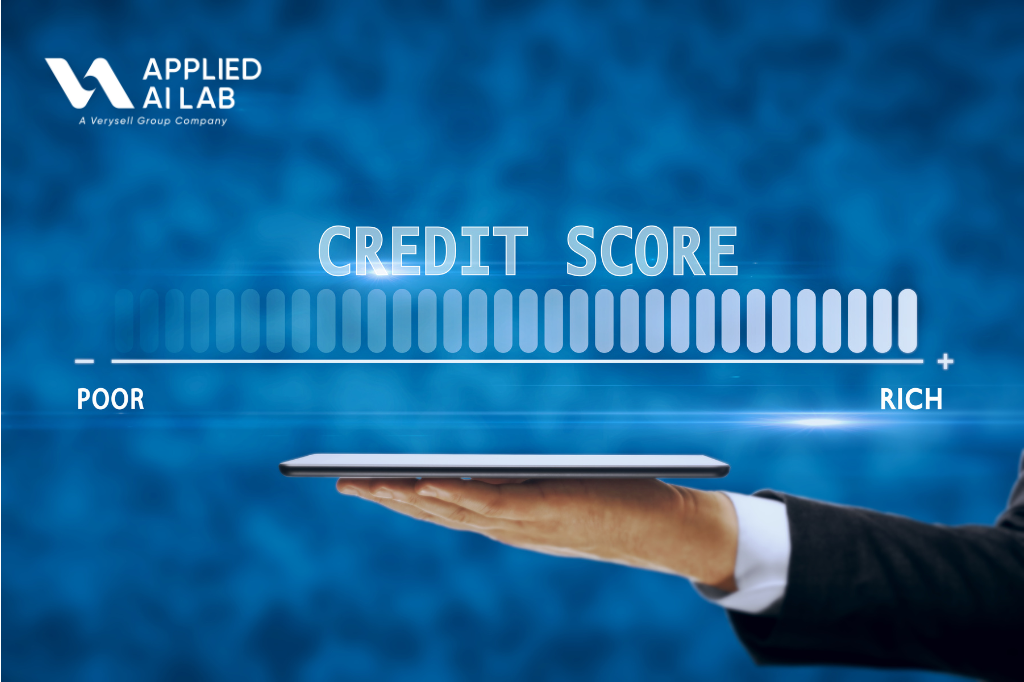
Business Understanding
The central aim of Credit Scoring Analysis is to distinguish between clients who are likely to fulfill their financial obligations and those at risk of default. The accuracy and predictive power of credit scoring empower banks to make informed lending decisions, channeling resources toward clients with a proven track record of creditworthiness. This not only minimizes the risk of default but also ensures the efficient allocation of resources, fostering a healthier financial ecosystem. As a result, banks can confidently expand their lending portfolios, support economic activities, and catalyze overall growth, thus fortifying the foundation of the finance sector and contributing to a more resilient economy.
In today’s landscape, banks dedicate substantial resources to gather comprehensive client data and assess creditworthiness, as traditional reliance on a scarcity of expert inspectors has become inadequate.
The accuracy and predictive power of credit scoring empowers banks to minimize risk and ensures the efficient allocation of resources, fostering a healthier financial ecosystem.
AI Solution
- Credit scoring analysis involves collecting a lot of historical data. This data comes from files that have information about loans, like credit scores, loan amounts, and more. There’s another set of data that shows how people paid back their debts over time.
- Before we use this data to predict credit scores, we clean it up and get it ready. It’s like getting a messy room organized before starting a project. Once the data is ready, we use computer programs called AI models to make predictions. These models are like smart tools that can understand patterns in the data. Some of these tools are called random forest, xGBoost, ensemble models, CNN, and RNN.
- These AI models help us make sense of the data. They work together like a team of musicians playing different instruments to create a beautiful piece of music. The main prediction we’re trying to make is the credit score, which tells us how trustworthy someone is to pay back loans.
- So, credit scoring analysis is like solving a puzzle with lots of data pieces. We clean the data, use AI models to understand it, and finally, we predict credit scores to help make better decisions about lending money.
Credit Scoring Processing Flow
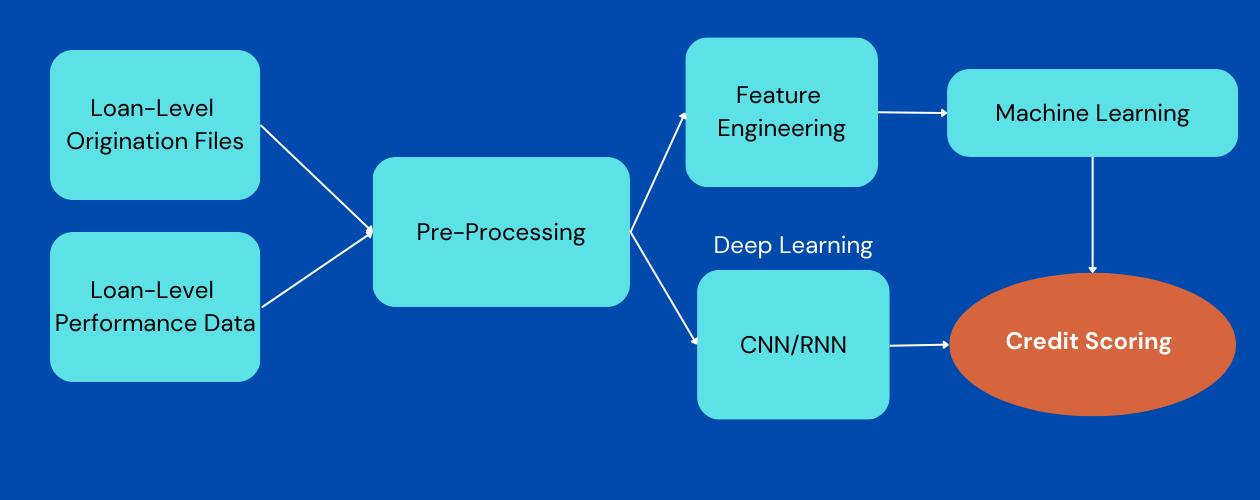
Expected Outcome
In the realm of finance, the predictive power of AI in credit scoring shines bright. Accurate credit score predictions serve as a catalyst for banks to amplify their revenue by a noteworthy 5% to 15%. Simultaneously, the shadow of credit loss is diminished, shrinking by an impressive margin of 20% to 40%. As an additional boon, the implementation of AI-driven solutions brings about a surge in efficiency, a surge ranging from 20% to 40%. This surge is owed to the streamlined automation of data extraction and the strategic prioritization of cases. Thus, the synergy between AI and credit scoring not only charts a path towards smarter lending decisions but also unveils avenues to bolster banks’ financial well-being and operational prowess.