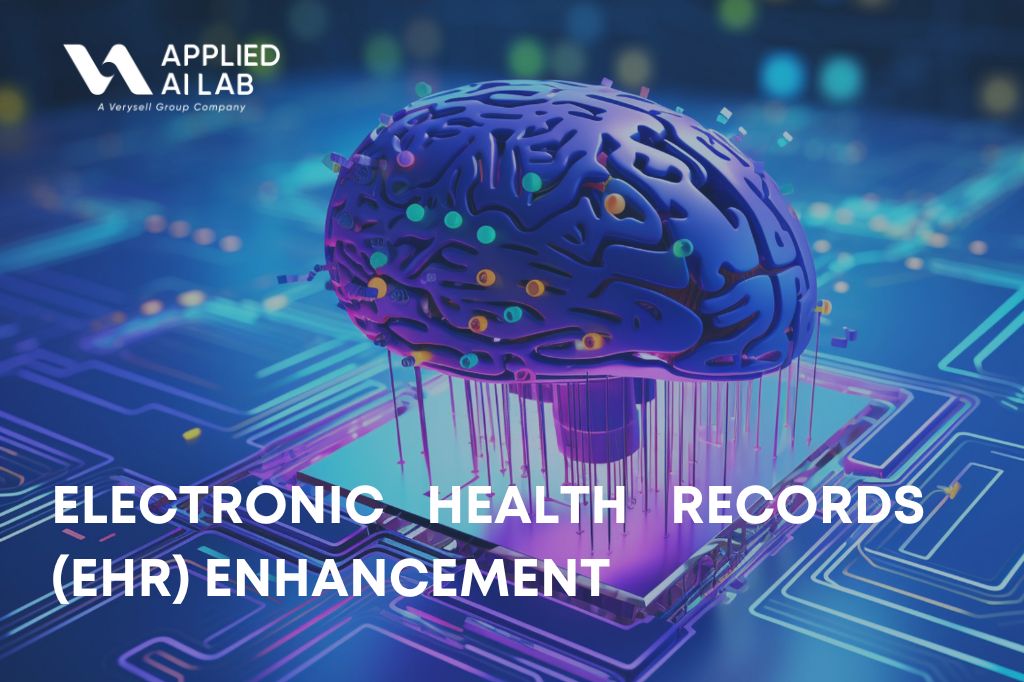
Business Challenges
The healthcare industry heavily relies on Electronic Health Records (EHR) systems to manage patient data efficiently. EHR systems digitize patient information, providing healthcare professionals with access to comprehensive medical histories, treatment plans, and clinical notes. In today’s healthcare landscape, EHR systems play a crucial role in improving patient care quality, enhancing communication among healthcare providers, and streamlining administrative processes. Efficient EHR systems facilitate timely access to patient information, enabling healthcare professionals to make informed decisions and deliver personalized care. Despite the benefits of EHR systems, healthcare organizations often face challenges related to clinical documentation.
Lengthy, unstructured clinical notes can hinder workflow efficiency, result in incomplete records, and pose challenges for data analysis and interpretation.
AI Solution
The implementation of AI-driven solutions in EHR systems involves several key components. An AI model, such as a Large Language Model (LLM), is trained on a large corpus of anonymized clinical notes and structured data elements. This model incorporates medical terminology, clinical context, and note-taking patterns to enhance the efficiency and accuracy of clinical documentation. Data used for training AI models is sourced from various departments within the healthcare organization, ensuring a diverse range of clinical scenarios and documentation styles are represented. Before training, the data is anonymized and cleaned to remove any sensitive or personally identifiable information.
During data preparation, clinical notes are structured and standardized to facilitate AI analysis. This involves cleaning, organizing, and categorizing clinical data elements to ensure consistency and accuracy in documentation. Through iterative training processes, AI models learn to recognize patterns, extract relevant information, and generate meaningful insights from clinical notes. These techniques enable AI models to understand and interpret complex medical language, extract relevant information, and generate actionable insights to support clinical decision-making.
Expected Outcome
AI-driven solutions address the challenges associated with clinical documentation by offering innovative features integrated into EHR systems. These features enhance workflow efficiency, improve documentation accuracy, and support clinical decision-making processes. By leveraging AI-powered features such as real-time note suggestion, automatic summarization, and standardized coding, the solution enhances clinical documentation efficiency and accuracy. Clinicians can now spend less time on documentation tasks, resulting in more time for direct patient care.
The benefits of AI implementation in EHR systems yield several benefits for healthcare organizations. Clinicians report a 20% reduction in time spent writing notes, allowing more time for direct patient care. AI-powered features streamline documentation processes, reduce turnaround time, and enhance overall workflow efficiency. Moreover, the percentage of complete notes increases by 15%, ensuring comprehensive documentation of clinical encounters. AI-driven features facilitate structured and standardized documentation practices, leading to more accurate and meaningful patient records. Additionally, automatic coding achieves 90% accuracy compared to manual coding, reducing errors and streamlining billing processes. AI-powered features extract relevant information from clinical notes and suggest appropriate medical codes, improving coding accuracy and efficiency.
Key performance indicators (KPIs) such as documentation turnaround time, note completion rates, and coding accuracy are used to measure the success of AI implementation. Healthcare organizations track these metrics to assess the impact of AI-driven solutions on workflow efficiency and patient care quality. The success of AI implementation is measured by improvements in workflow efficiency, documentation accuracy, and coding accuracy. Healthcare organizations observe tangible benefits such as reduced documentation time, increased note completion rates, and improved billing processes.
While AI plays a central role in enhancing clinical documentation processes, human oversight and input remain essential for ensuring the quality and relevance of patient records. AI augments the capabilities of healthcare professionals by automating routine documentation tasks, allowing them to focus more time and attention on direct patient care. AI-powered features within EHR systems enhance the capabilities of healthcare professionals by providing real-time assistance with documentation tasks. Clinicians can leverage AI-driven suggestions and summaries to improve the efficiency and accuracy of clinical documentation, ultimately leading to better patient care outcomes. The integration of AI into EHR systems has positive implications for the healthcare industry as a whole. By enhancing workflow efficiency, improving documentation accuracy, and supporting clinical decision-making processes, AI-driven EHR enhancements contribute to the growth and innovation of the healthcare industry. Healthcare organizations that adopt AI-powered EHR solutions gain a competitive edge by improving patient care quality, reducing administrative burden, and enhancing overall efficiency. AI adoption fosters innovation and drives positive outcomes for both healthcare providers and patients.