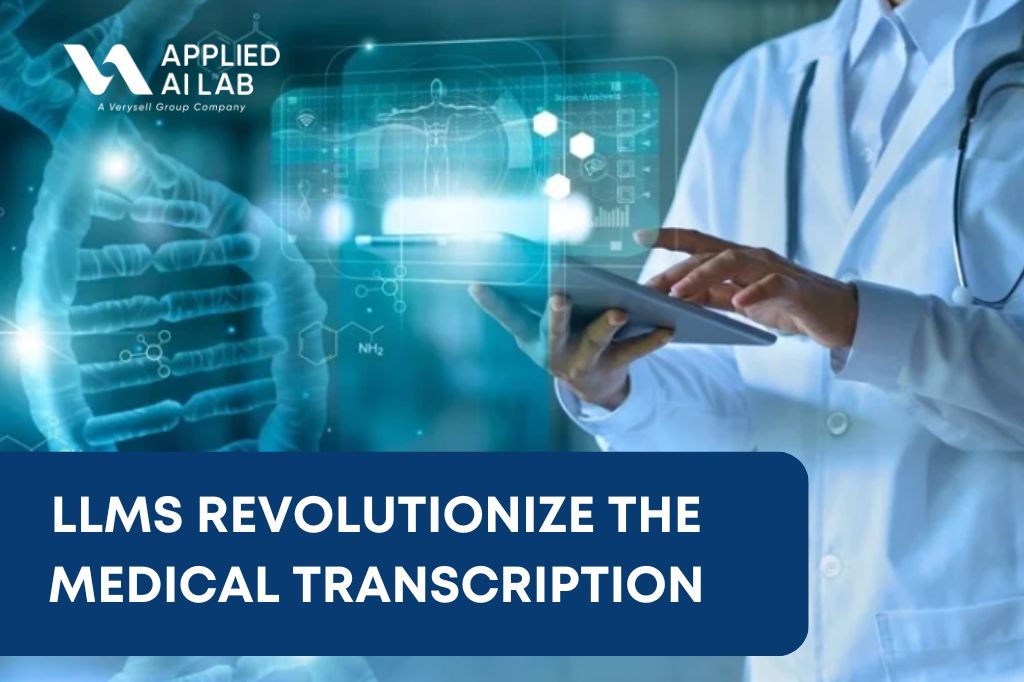
Business Challenges
Medical transcription is an essential component of healthcare documentation, involving the conversion of spoken medical narratives into text reports. In today’s healthcare landscape, accurate and efficient transcription is crucial for maintaining patient records, facilitating communication among healthcare professionals, and ensuring quality patient care. However, traditional transcription methods often struggle with the complexity of medical terminology and accents, leading to errors and inefficiencies.
AI Solution
The implementation of AI-driven solutions, specifically Large Language Models (LLMs), offers a transformative approach to medical transcription. These models are trained on large datasets of anonymized medical audio recordings and transcribed reports, enabling them to understand and accurately transcribe complex medical language. By leveraging advanced natural language processing (NLP) techniques, LLMs can effectively interpret medical terminology, accents, and speech patterns, leading to improved transcription accuracy and efficiency.
The data used for training LLMs is sourced from various departments within the hospital, ensuring a diverse range of medical scenarios and accents are represented. Before training, the data is anonymized and cleaned to remove any sensitive or personally identifiable information. During training, the LLM learns to associate audio inputs with corresponding transcribed text outputs, gradually improving its accuracy and performance through iterative optimization algorithms.
The integration of LLMs with existing transcription systems allows healthcare providers to dictate their notes directly into the system, which then generates accurate transcripts in real-time. This seamless integration streamlines the transcription process, reduces turnaround time, and enhances overall efficiency.
Expected Outcome
The implementation of AI-powered medical transcription yields several benefits for healthcare providers and patients alike. By reducing errors and streamlining the transcription process, AI leads to significant improvements in efficiency, with a 30% reduction in transcription turnaround time. These efficiency gains translate into cost savings for hospitals, as fewer resources are required for manual transcription and error correction.
Moreover, the accurate and timely transcription provided by AI enhances decision-making by providing healthcare providers with access to up-to-date and comprehensive patient records. Metrics such as transcription accuracy rates, turnaround time, and user satisfaction are used to measure the success of the AI implementation.
While AI plays a central role in improving transcription accuracy and efficiency, human oversight and input remain crucial for ensuring the quality and relevance of transcribed reports. AI augments the capabilities of healthcare providers by automating routine transcription tasks, allowing them to focus more time and attention on patient care.
In conclusion, the integration of Large Language Models (LLMs) into the medical transcription process represents a significant advancement in healthcare documentation and communication. By leveraging AI technologies, healthcare providers can improve transcription accuracy, efficiency, and overall patient care quality, ultimately leading to better outcomes for both patients and providers.