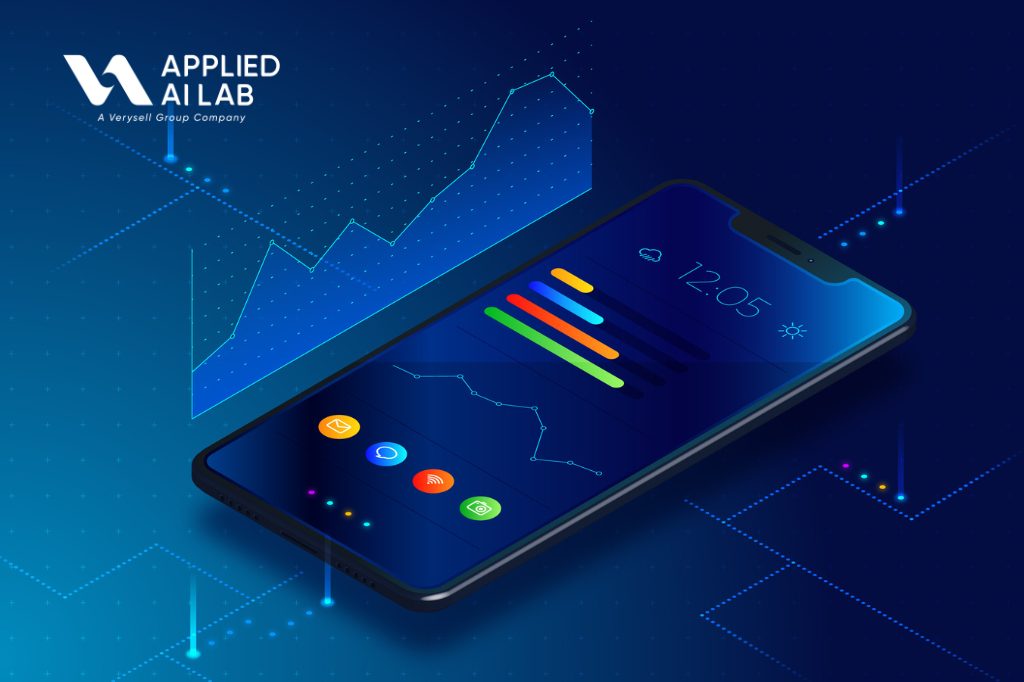
Business Understanding
In the dynamic realm of 5G, network operators are presented with a unique opportunity to dynamically craft and deploy a diverse range of services, such as massive IoT, mMTC, and eMBB. Yet, this heightened flexibility and complexity necessitate solutions that uphold security, reliability, and resource allocation in a dynamically robust and dependable manner. Here, the pivotal role of AI technology comes into play, serving as the cornerstone that empowers the future networks, encompassing 5G and beyond.
Three distinct domains emerge as the arena where AI technology reshapes the landscape: Network Planning, Network Diagnostics and Insights, and Network Optimization and Control. Two pivotal challenges underscore this transformation. Firstly, the endeavor of placing probes at every network point for data collection becomes impractical. Secondly, the massive and sparse operational data, a treasure trove of insights, poses a formidable challenge for human experts to digest effectively, given the limitations of traditional theoretical and empirical processing.
Amidst the surging demands of transportation, smart cameras, media streaming, and beyond, the accurate prediction of data traffic volume emerges as a linchpin for Service Providers to honor their Service Level Agreements. Yet, the intricacies of dense urban environments and the ever-shifting landscape of mobile traffic due to user mobility render the placement of probes complex and expensive. In this intricate dance of variables, it becomes evident that AI technology stands as the sole reliance for 5G and future Networks, supplanting human expertise and steering the course towards transformative possibilities.
AI Solution
- To surmount these complexities, a promising avenue lies in the adoption of a Deep Spatio-Temporal Network (D-STN).
- The architecture involves leveraging 3D convolution layers to discern the intrinsic learnable features inherent in spatio-temporal traffic data. This process effectively unravels the underlying patterns that govern the movement of data across space and time.
- Further enhancing the predictive prowess, Convolution Long Short Term Memory (ConvLSTM) units come into play. By integrating the strengths of both convolutional and LSTM structures, these units facilitate accurate and dependable long-term projections of mobile traffic patterns.
- To round off the model’s capabilities, Fully Connected Layers are employed. These layers function as the final touchpoint, effectively regressing the projected traffic volume. In doing so, they translate the complex web of learnings and predictions into a comprehensive and actionable forecast.
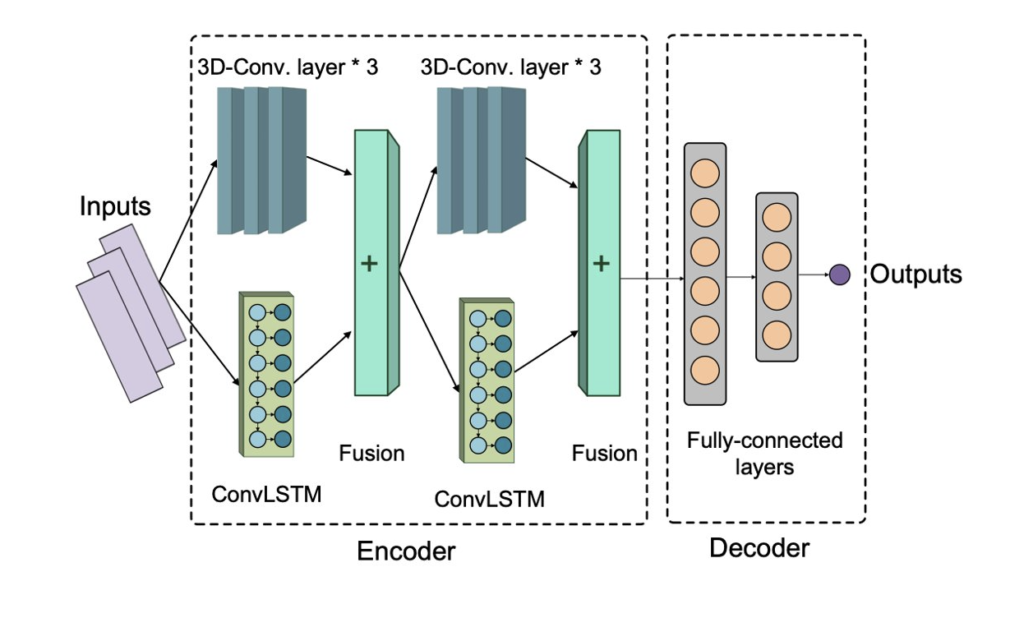
Traffic volume in one cell forecast over 10 hours (after 2-hour observations) with Holt-Winters exponential smoothing, ARIMA, and D-STN, which captures spatio-temporal correlations .
Mobile Traffic Forecasting for one cell
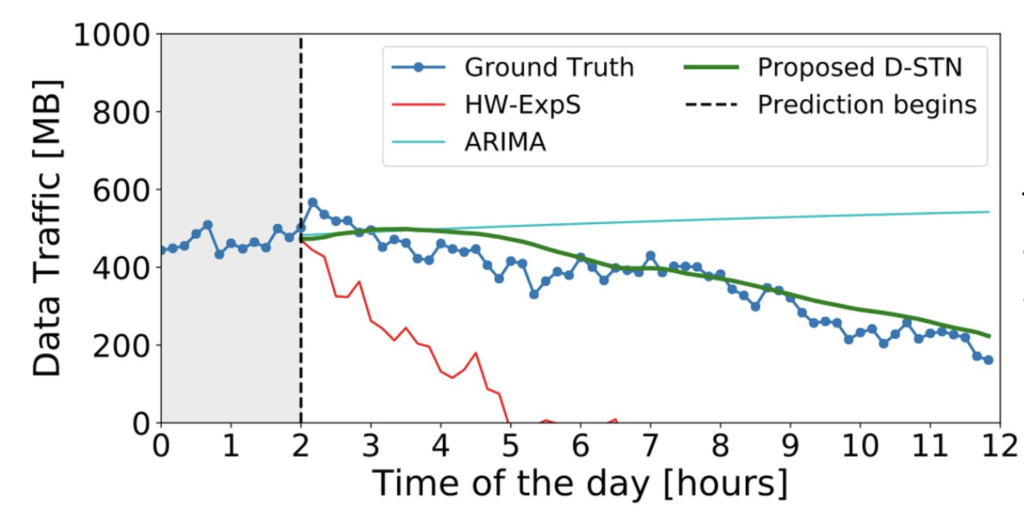
Expected Outcome
In the realm of Mobile Traffic Forecasting, the fusion of AI technology with the intricate dynamics of data holds transformative potential. The utilization of a Deep Spatio-Temporal Network (D-STN) stands as a beacon of innovation, effectively addressing the complex challenges that mobile traffic prediction poses. With the strategic deployment of 3D convolution layers, Convolution Long Short Term Memory (ConvLSTM) units, and Fully Connected Layers, the AI-driven framework becomes a powerful predictive tool. It discerns hidden patterns within spatio-temporal traffic data, crafts reliable long-term projections, and ultimately regresses accurate traffic volume predictions. Through this amalgamation of cutting-edge AI methodologies, Mobile Traffic Forecasting transcends conventional limitations, offering a sophisticated and adaptable solution that paves the way for smarter, data-driven decision-making in the fast-evolving landscape of mobile networks.