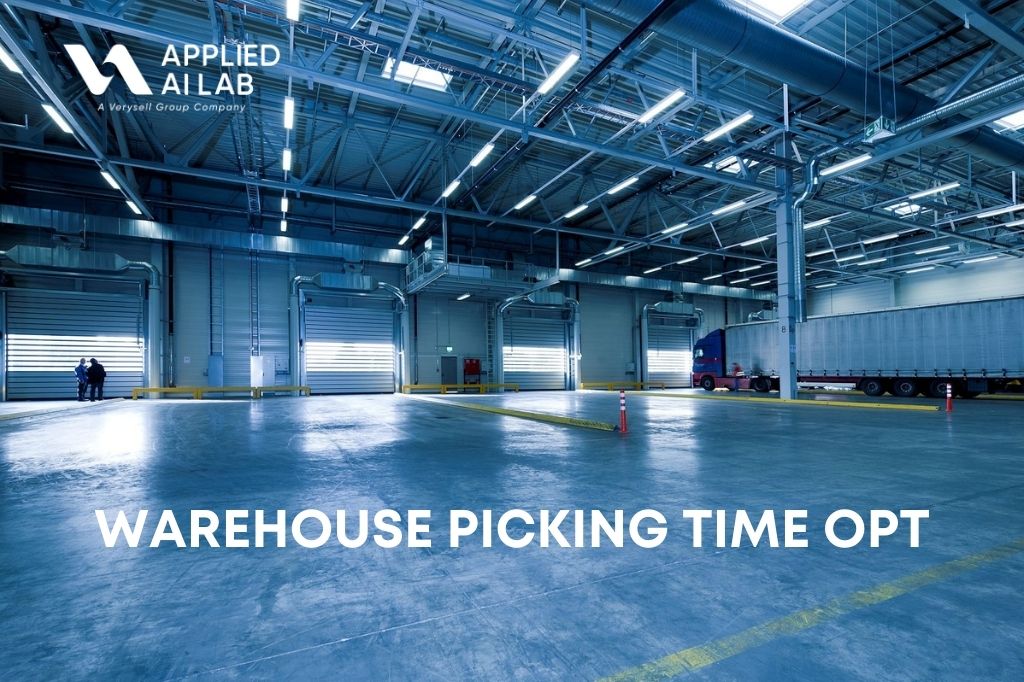
Business Challenges
Retailers are embracing the transformative potential of Data Analytics to redefine their warehouse picking processes, fundamentally reshaping their ability to meet customer demands effectively.
Warehouses, the central hubs for storing and distributing products, serve as the backbone of the supply chain and play a pivotal role in the success of retail operations. These warehouses are typically organized into fixed layout zones that are rarely altered. However, this static structure poses a significant challenge as market demands for goods are in constant flux. Consequently, the efficiency of the picking process within these warehouses becomes compromised, resulting in operational bottlenecks.
One of the primary challenges in enhancing warehouse efficiency lies in optimizing the layout to minimize picking time. In the dynamic realm of retail, where time equates to money, the utilization of Data Analytics is essential. By employing advanced analytics techniques, retail companies can analyze historical order data, inventory flow, and customer demand patterns. This data-driven approach enables them to strategically reconfigure their warehouse layouts, ensuring that frequently requested items are positioned for swift access.
The application of Warehouse Picking Time Optimization through Data Analytics not only mitigates operational bottlenecks but also empowers retailers to meet customer expectations promptly. This, in turn, contributes to heightened customer satisfaction and provides a competitive edge in the ever-evolving retail landscape. By leveraging Data Analytics, retailers can not only adapt to changing market demands but also proactively optimize their operations, ultimately revolutionizing their approach to fulfilling customer needs.
AI Solution
To address this challenge, a solution was devised that leveraged historical picking data and AI techniques:
- Historical Data Analysis: The dynamically changing demands for goods in the market are reflected in the historical picking data of the warehouses. This data analysis phase extracts insights about the most demanding goods and their associated picking times. It reveals inefficiencies in the current warehouse operations.
- Standardized Operations: It is assumed that goods are moved within the warehouse using either forklifts or robots. The times required for traveling, loading, and picking are standardized based on this assumption.
- Reinforcement Learning Model: A reinforcement learning model is implemented to reorganize the layout of warehouses with the objective of minimizing picking time. The model uses historical picking data from the warehouses to train and refine its decision-making capabilities.
Expected Outcome
The anticipated outcome of implementing the reinforcement learning model is substantial:
- Optimized Layout: The results generated by the reinforcement learning model reveal that the most in-demand goods should be strategically positioned near the unloading gates of the warehouses. This layout adjustment is projected to reduce picking time by approximately 30%.
By optimizing the layout of warehouses to align with changing market demands, this solution not only enhances operational efficiency but also contributes to significant time and cost savings in the warehousing process.